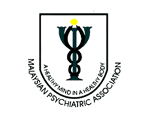
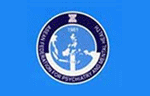
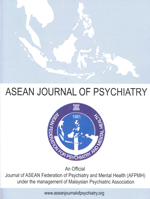
Google Scholar citation report
Citations : 5373
ASEAN Journal of Psychiatry received 5373 citations as per google scholar report
ASEAN Journal of Psychiatry peer review process verified at publons
Journal Name | ASEAN Journal of Psychiatry (MyCite Report) | ||||
---|---|---|---|---|---|
Total Publications | 456 | ||||
Total Citations | 5688 | ||||
Total Non-self Citations | 12 | ||||
Yearly Impact Factor | 0.93 | ||||
5-Year Impact Factor | 1.44 | ||||
Immediacy Index | 0.1 | ||||
Cited Half-life | 2.7 | ||||
H-index | 30 | ||||
Quartile |
|
- Anxiety Disorders
- Behavioural Science
- Biological Psychiatry
- Child and Adolescent Psychiatry
- Community Psychiatry
- Dementia
- Community Psychiatry
- Suicidal Behavior
- Social Psychiatry
- Psychiatry
- Psychiatry Diseases
- Psycho Trauma
- Posttraumatic Stress
- Psychiatric Symptoms
- Psychiatric Treatment
- Neurocognative Disorders (NCDs)
- Depression
- Mental Illness
- Neurological disorder
- Neurology
- Alzheimer's disease
- Parkinson's disease
Abstract
INTELLIGENT HANDHELD EXPERT SYSTEM (HES) FOR DIAGNOSIS OF AUTISM SPECTRUM DISORDER AND ITS SEVERITY LEVEL
Author(s): Vikas Khullar, Harjit Pal Singh, Manju BalaObjective: Autism Spectrum Disorder (ASD) is a complex neurological developmental disorder that could be diagnosed early usually before the age of 3 years and the diagnosis is the most important determining factor for the treatment of ASD. The aim of present work is to design and implement a Handheld Expert System (HES) based on Diagnostic and Statistical Manual of Mental Disorder, fifth edition (DSM-V) for the diagnosis and severity assessment of ASD. The hand-held device was trained by artificial neural network to correctly diagnosis ASD and identifies its severity level. Methods: The learning of HES for ASD diagnosis was performed by a back propagation neural network algorithm with data set created based on DSM-V. The ability of Artificial Intelligence (AI) based HES was measured in terms of epochs, training/testing data, and statistical stability on the basis of accuracy, losses, mean squared error, and execution time to validate the performance of the system. The HES was designed to consume less training/testing time with more efficient and accurate AI approach. The stability of HES was validated for the data set of 40 ASD and Typically Developed (TD) subjects (20 ASD and 20 TD). Results: The implementation of HES for diagnosis of 40 subjects (20 ASD and 20 TD) based on the proposed expert system has provided 100% accuracy in reference with DSM-V. The results were also validated by statistical analysis. Conclusion: Since AI based HES for diagnosis of ASD and determination of its severity provided accurate results in reference to DSM-V criteria, the possibility of the use of proposed HES for diagnosis of ASD is very high.